LangChain vs Auto-GPT vs BabyAGI: A Comparative Analysis of Open-Source AI Agent Frameworks

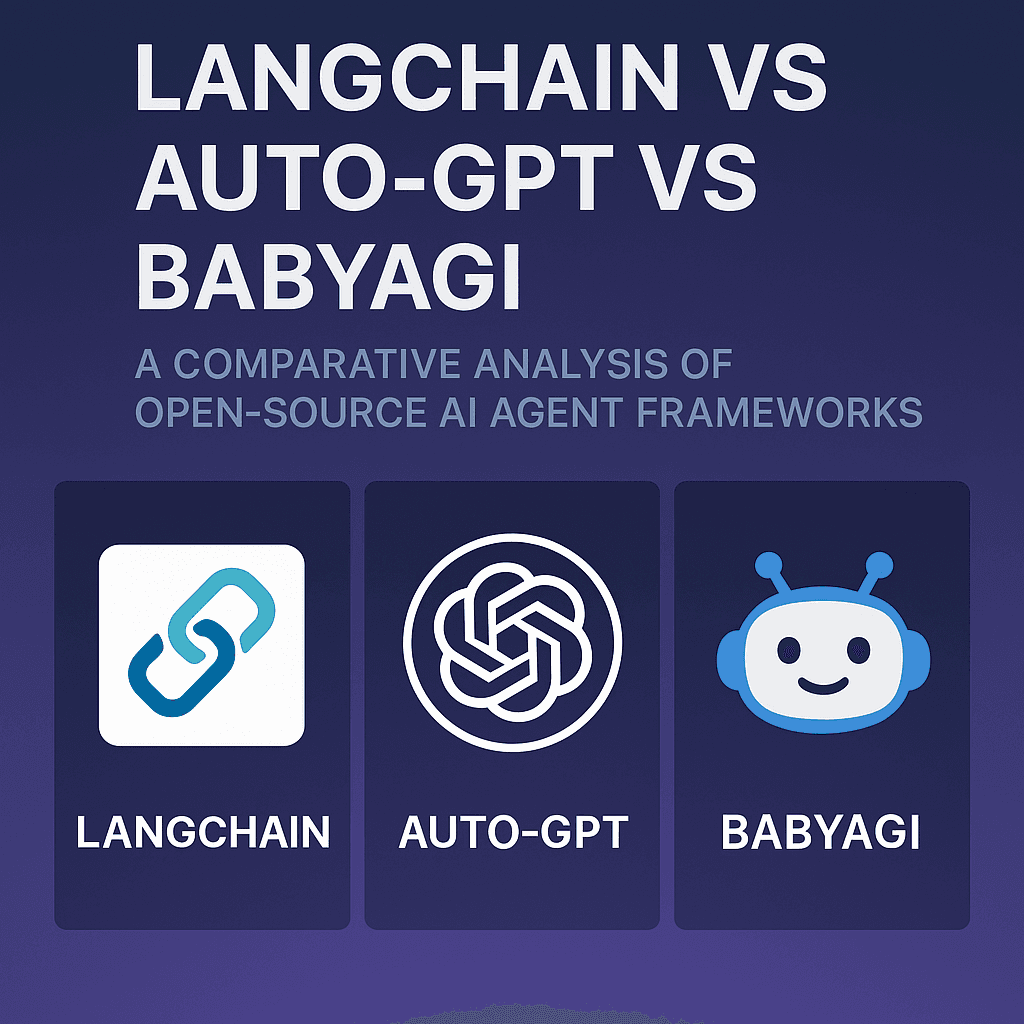
The emergence of autonomous agents—AI systems capable of independently executing multi-step tasks—represents a pivotal advancement in applied artificial intelligence. Enabled by large language models (LLMs), these agents exhibit the ability to plan, reason, utilize tools, and adapt based on environmental feedback.
Several open-source frameworks have been developed to support the implementation of such agents. Among the most prominent are LangChain, Auto-GPT, and BabyAGI. While each serves the overarching goal of facilitating autonomous behavior, their underlying architectures, intended use cases, and degrees of complexity differ considerably.
This article offers a comparative evaluation of these frameworks to support developers, researchers, and organizations in selecting the most appropriate tool for their specific application domains.
Understanding Autonomous AI Agents
Autonomous AI agents are computational systems designed to perform complex tasks with minimal human intervention. These agents interact with digital environments, make informed decisions, and execute actions aligned with high-level objectives.
Unlike conventional automation tools, agents powered by LLMs possess the capacity to:
- Interpret and deconstruct natural language instructions
- Generate sub-tasks dynamically
- Prioritize and execute tasks iteratively
- Access external tools, APIs, and knowledge sources
- Maintain contextual continuity via memory modules
Frameworks such as LangChain, Auto-GPT, and BabyAGI offer architectural scaffolding to operationalize these capabilities in real-world applications.
1. LangChain: A Modular Framework for LLM-Powered Applications
LangChain is a comprehensive, extensible framework that facilitates the integration of LLMs into complex software systems. It provides modular abstractions—such as agents, chains, tools, and memory—that enable the composition of sophisticated reasoning workflows.
Key Capabilities
- Integration with major LLM providers (e.g., OpenAI, Cohere, Anthropic)
- Support for various memory backends and vector databases (e.g., FAISS, Pinecone)
- Advanced agent strategies (e.g., ReAct, Plan-and-Execute)
- Interfacing with tools including web search, APIs, file systems, and code interpreters
Strengths
- High degree of customization and composability
- Suitable for production environments and enterprise-scale deployments
- Robust documentation and vibrant open-source community
Considerations
- Initial learning curve due to its architectural complexity
- Requires thoughtful orchestration of components for advanced use cases
Ideal Use Case
LangChain is most appropriate for developers and research teams seeking to construct scalable, robust, and customizable AI agent systems.
2. Auto-GPT: Autonomous Goal-Oriented Task Execution
Auto-GPT is an experimental open-source framework designed to showcase the autonomous potential of LLMs. It operates by recursively generating and executing prompts to achieve high-level goals without further human input.
Key Capabilities
- Recursive task planning and self-prompting
- File system interaction and memory persistence
- Plugin support for external APIs and tools
- Simple CLI-based interface for deployment
Strengths
- Enables hands-off, fully autonomous task execution
- Demonstrates emergent problem-solving capabilities of LLMs
- Requires minimal setup for initial experimentation
Considerations
- Prone to logical inconsistencies and infinite loops
- Not designed for high-stakes or real-time use
- Limited transparency in internal decision processes
Ideal Use Case
Auto-GPT is particularly suitable for experimental research, prototyping, and demonstrations of goal-directed LLM behavior.
3. BabyAGI: A Minimalist Learning Loop for Task Management
BabyAGI is a simplified agent loop that simulates a process of task prioritization, execution, and regeneration. It is designed primarily for conceptual clarity and educational experimentation.
Key Capabilities
- Lightweight task queue and execution loop
- Dynamic generation of sub-tasks
- Integration with vector stores for contextual memory
Strengths
- Extremely simple to understand and extend
- Ideal for conceptual prototyping and didactic purposes
- Minimal system requirements and fast iteration cycles
Considerations
- Not production-ready
- Lacks support for complex logic and error handling
- Ineffective for large-scale or high-reliability use cases
Ideal Use Case
BabyAGI is best employed for educational purposes, learning exercises, or lightweight prototypes requiring iterative reasoning.
Comparative Summary
Feature | LangChain | Auto-GPT | BabyAGI |
---|---|---|---|
Architecture | Modular, composable | Recursive, autonomous | Task queue with feedback |
Customization | High | Moderate | Low |
Ease of Use | Moderate | High | Very High |
Production Readiness | Yes | No | No |
Tool and API Integration | Extensive | Moderate | Minimal |
Memory Capabilities | Advanced | Basic | Basic |
Primary Use Case | Scalable applications | Goal-driven experimentation | Learning and concept testing |
Ideal Users | Engineers, researchers | Innovators, hobbyists | Students, educators |
Selecting the Right Framework
The optimal choice among LangChain, Auto-GPT, and BabyAGI depends on several key factors:
- Choose LangChain for enterprise or research-grade systems that demand flexibility, reliability, and scalability.
- Choose Auto-GPT for proof-of-concept exploration where full autonomy and rapid iteration are desired.
- Choose BabyAGI for educational environments or when a minimal agent loop suffices for experimentation.
In practice, hybrid approaches can also be advantageous. For example, LangChain’s agent orchestration can be combined with BabyAGI’s task queue model to produce more flexible and traceable agent behavior.
Conclusion
Autonomous AI agents represent a significant advancement in the field of artificial intelligence, offering the potential to revolutionize knowledge work, automation, and decision-making. While LangChain, Auto-GPT, and BabyAGI offer distinct approaches to agent design and deployment, they collectively highlight the creative possibilities of integrating LLMs into dynamic, goal-driven systems.
As the technology matures, we anticipate increased convergence and cross-pollination among these frameworks, paving the way for more adaptive, transparent, and generalizable AI agents.
References
- LangChain Documentation. https://docs.langchain.com
- Auto-GPT GitHub Repository. https://github.com/Torantulino/Auto-GPT
- BabyAGI GitHub Repository. https://github.com/yoheinakajima/babyagi
- GPT-4 Technical Report. https://openai.com/research/gpt-4